Research
Our primary research goal is to understand how visual information is represented by the visual system and how it is encoded and integrated into memory.
What constraints do visual processing and prior knowledge impose on information encoding?
How do visual representations transform from perception to working memory to long-term memory?
How do we store information about items that are strongly related to other items or to the context?
Our research approach draws on formal models to understand the common ground between vision and memory. In particular, we develop behavioral and sometimes EEG or fMRI paradigms designed to tap what information is represented and what information persists in visual memory, and use signal detection-based, Bayesian, connectionist and information theoretic tools to formalize the underlying memory representations. Our research has focused on three core areas: the representations involved in visual working memory and visual long-term memory; the nature of our existing knowledge of objects and scenes; and what kinds of subtle statistical regularities observers learn from the world.
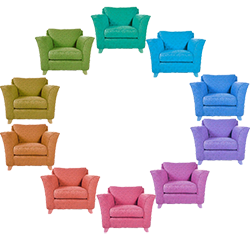
The structure and capacity of visual long-term memory
One major concentration of our research has been investigating the structure of information storage and capacity of long-term memory for visual information. In particular, we have focused our research not on how many items observers can remember, but what they remember about those items and what might support this massive visual long-term memory capacity. We have found evidence that long-term memory can not only store thousands of objects, but also can store those objects with a remarkable amount of visual detail. Furthermore, this capacity seems to be mediated by our knowledge about these objects: the more conceptually distinct an object or scene, the better we are able to remember it. We have also examined the representation of visual stimuli in long-term memory using psychophysical methods, focusing on the fidelity of memory for particular features (like color), as well as the extent to which visual objects are stored in memory as single bound units versus independent, unbound properties.A Sample of Relevant Papers (see Publications page for more)
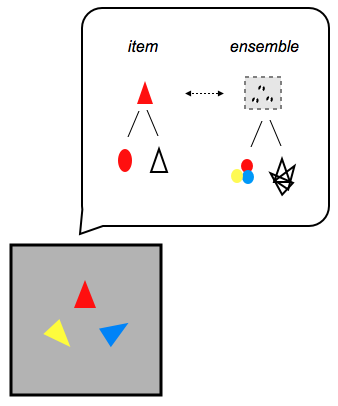
The structure of visual working memory
When we perceive the visual world, we bring to bear prior knowledge about the things we see. How does such knowledge affect the amount of visual information we can hold in mind at once? Nearly all measures of working memory capacity have assumed that capacity is independent of prior knowledge, and have attempted to quantify how many items observers can remember (e.g., we are limited to 4 items). Rather than assuming observers' representations are based on the storage of a fixed number of independent items, we have investigated working memory capacity from a constructive memory perspective: In contrast to the predictions of existing models, we have found that observers take advantage of prior knowledge when representing a display in working memory, and represent the display hierarchically - that is, they encode structured representation consisting of not only individual items but also a summary or visual texture of the display.A Sample of Relevant Papers (see Publications page for more)
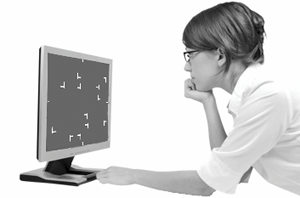
Statistical learning and effects of exposure
Another concentration of our research has been investigating our ability to learn statistical regularities from the world. We've investigated this in two contexts. The first is visual search: We frequently search for objects during our everyday lives, and the scene we find ourselves in helps predict where the object we are searching for will be located. We've investigated what information we extract and remember as we perform visual search tasks, particularly what we learn by performing a search over and over again in the same context (contextual cueing), and what we learn as we perform a particular search (rapid resumption).In addition, we've investigated people's ability to learn more high-level regularities, like complex temporal sequences. We've shown that people are sensitive to statistical regularities in the order stimuli appear: we learn both the order of particular items and of higher-level patterns, like the order of the semantic categories of the stimuli. Furthermore, we seem to do this almost entirely implicitly. Learning at the level of semantic categories probably helps us compress the amount of information we need to store, since any regularity we learn applies to many possible individual items.
Relevant Papers
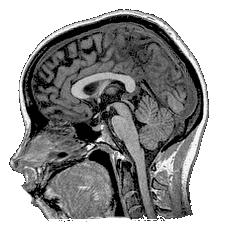
Scene representation and spatial ensembles
Human scene categorization is remarkably rapid and accurate. But what allows this rapid recognition and what do we ultimately extract from an image of a visual scene?
We have examined this question from a number of different angles. For example, we have shown that observers implicitly learn that certain kinds of scenes tend to follow each other; and that memory performance with scenes is nearly identical to performance with objects, suggesting scenes and objects are treated at similar levels of abstraction in memory. Most recently, we've examined the neural correlates of scene representation. What determines the brain's representation of a scene -- is it a combination of objects that constitute a scene (e..g, whether the scene contains trees or buildings) or is it the spatial structure or geometry of the space? (e.g., whether the space is very open or closed).
Using fMRI multi-voxel pattern analysis, we have examined whether the neural representation of scenes reflect global properties of scene structure, such as the openness of a space, or properties of objects within a space, such as naturalness. Interestingly, in the PPA (a scene-selective brain region), we find a high degree of similarity between scenes with similar spatial structures; however, in the LOC (a region sensitive to objects), we find a high degree of similarity between scenes that contain similar objects. These results suggest that there are multiple visual brain regions involved in analyzing different properties of a scene.
We have also recently begun to address the relationship between summary statistics or ensemble perception and the recognition of real-world scenes. Are all ensembles processed using the same mechanisms? How does ensemble perception relate to texture perception and scene recognition?